- February 2, 2016
- Posted by: Phillip W. Duff
- Category: Latest Post
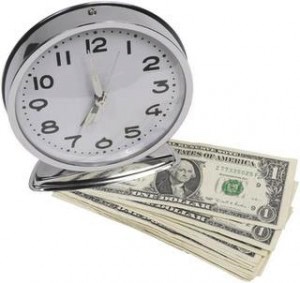
Debt settlement companies seem to fit into one of two categories when it comes to analytics. The first and more common are the smaller debt relief agencies that are working from a canned software package or a CRM designed for other business lines. The second are the very large companies that are working from propitiatory systems they have developed or built out to work within another system with much expense.
So let’s discuss first how the big guys use that fancy system to increase profits, reduce time to settlement and decide which debt is ripe and what is still being worked First, the big guys have data integrity. They have built the systems to accommodate the data in which they will be using later in the decision process. And, they have protocols in place to make sure the data is clean. In contrast, many smaller companies have dirty data from the intake process that continues to degrade to a point that it i no longer mineable.
For example, let’s explore the infomation taken from the consumer at enrollment, specifically the debt enrolled. The smarter companies have standardized their data so it’s consistent. The smaller guys see a Chase Air Mile card branded with Southwest Air logo and a Chase Aspire card the same way or actually in several analytic columns. The smaller company who has no standardization automation in their system will likely have those two accounts listed above entered into the system as the following options;
• Chase
• CHS
• Aspire
• Southwest card
• Chase SW
• CHASE CARD
And many more…
So, you can see how the data is now dirty and any decisions downstream in the settlement process made from that data are flawed. By having no standardization or standardization automation, the data is corrupted.
One of the next differences is that technology makes the big companies append data to the consumer’s record which help them make decisions. Some of the data is just more accurate data from a credit bureau or address updates, but also some of the data mimics what the debt collectors has appended to their file. Data such as: ;
• Fico score
• Home ownership
• Income per zip
• Employment status
• Days since charge off
Leave a Reply
You must be logged in to post a comment.